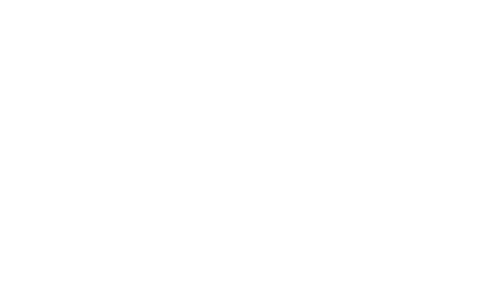
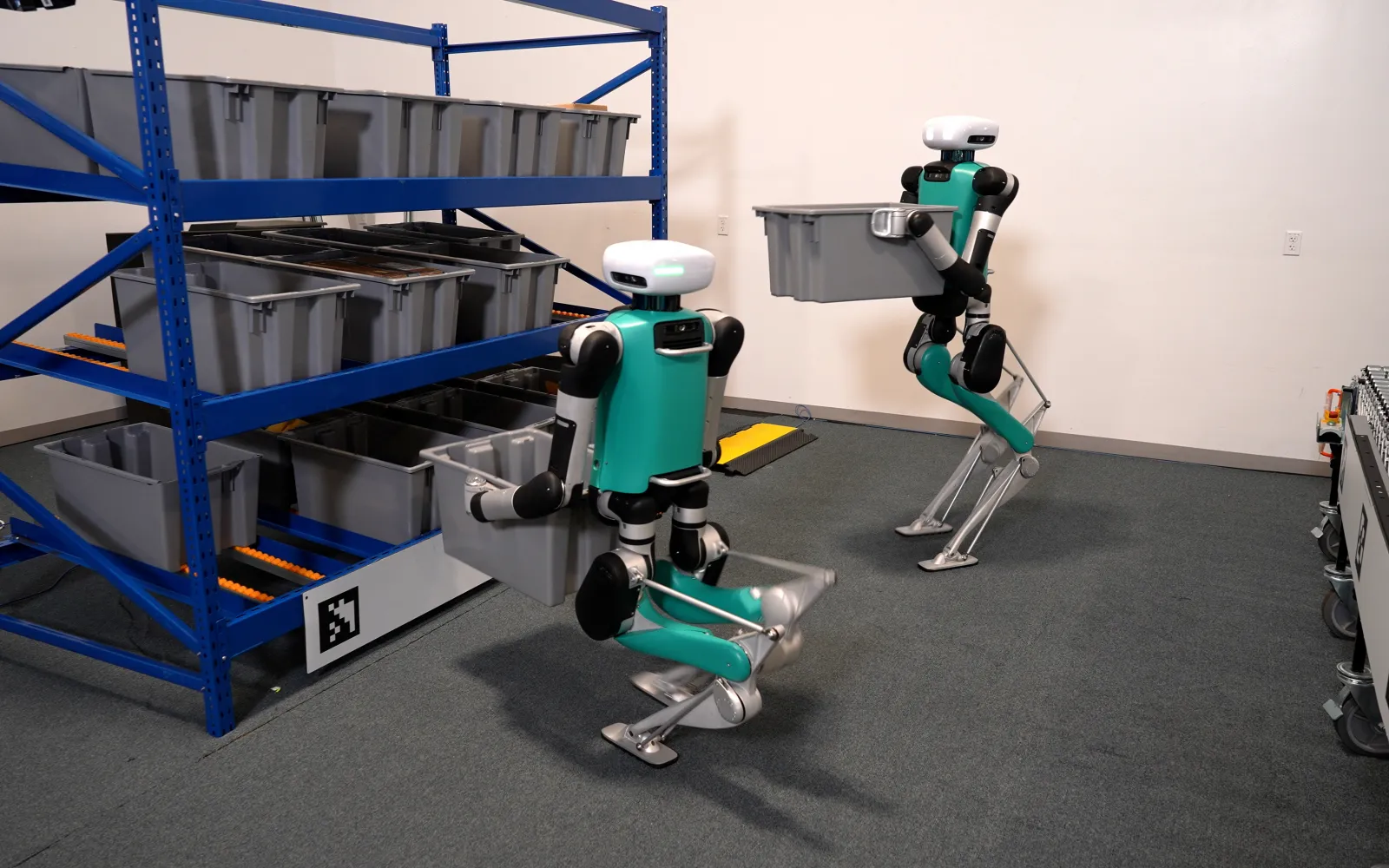
Agility Robotics
Building robots that let humans be more human
The 2024 edition of the DCVC Deep Tech Opportunities Report explains the guiding principles behind our investing and how our portfolio companies contribute to deep tech’s counteroffensive against climate change and the other threats to prosperity and abundance. Four of the opportunities described in the report relate to computing; this is the second.
Today’s most powerful foundation models, such as GPT‑4, learn mainly from the statistical patterns in vast amounts of data, but it’s a mistake to assume that data-fueled deep learning, by itself, can cut through every real-world problem. In deep tech, there are many domains where progress depends on a judicious mix of real-world data, physics understanding, and old-fashioned expert systems, painstakingly coded with rules and symbolic logic about the world. One of those domains is robotics.
Agility Robotics, which we’ve backed since 2020, makes and sells Digit, a flexible bipedal robot designed to work alongside humans in factories and distribution centers designed for humans. The company is currently testing Digit in partnership with Amazon Robotics for tasks like carrying containers, called totes, from shelves to conveyors and recycling empty containers. In recent demonstrations, Agility has shown that it’s possible to use a large language model to translate natural-language requests from human users into plans Digit can carry out. But these same experiments show the limitations of AI models on their own, says Melonee Wise, Agility’s chief product officer.
As a simple example, she points to the unique jargon of logistics. If an automated pick-and-place system puts the wrong item into a tote, that tote is sent to the “hospital” for correction. If an item needs to get on a truck for shipping today, it’s placed in a “hot” tote. “If we just threw all this data at the system, none of that would be contextualized,” Wise says. “The minute a manager says, ‘I have a load of hots coming in,’ everything’s going to break.”
Agility Arc, the company’s cloud-based automation management platform, is the place where customers can build in that kind of site-specific context. And it’s also the level where machine learning becomes truly integral to Agility’s system, Wise says, because Arc is a hybrid: a trainable expert system. Sensor data and other data flowing into the platform help Arc’s AI models learn, and they can then make better recommendations to customers about how to set up their operations.
“If you want to improve the throughput of a facility, you need an actual expert system that has the context of the problem you’re trying to solve,” Wise says. “And the only way we get that context is, one, by measuring the real world, and, two, understanding why Digit did something in the real world.”
“There is a danger that people will believe that because AI can see beyond the horizon of immediate human perception, it magically solves hard limits in physics and engineering,” DCVC managing partner Matt Ocko observes. “There are robotics companies saying we can rig up automata with mechanical tendons and AI is going to bring them to life. But if you haven’t done the engineering to make a robot actually work in human and industrial spaces — which Agility has — a large language model is not going to save you.”