
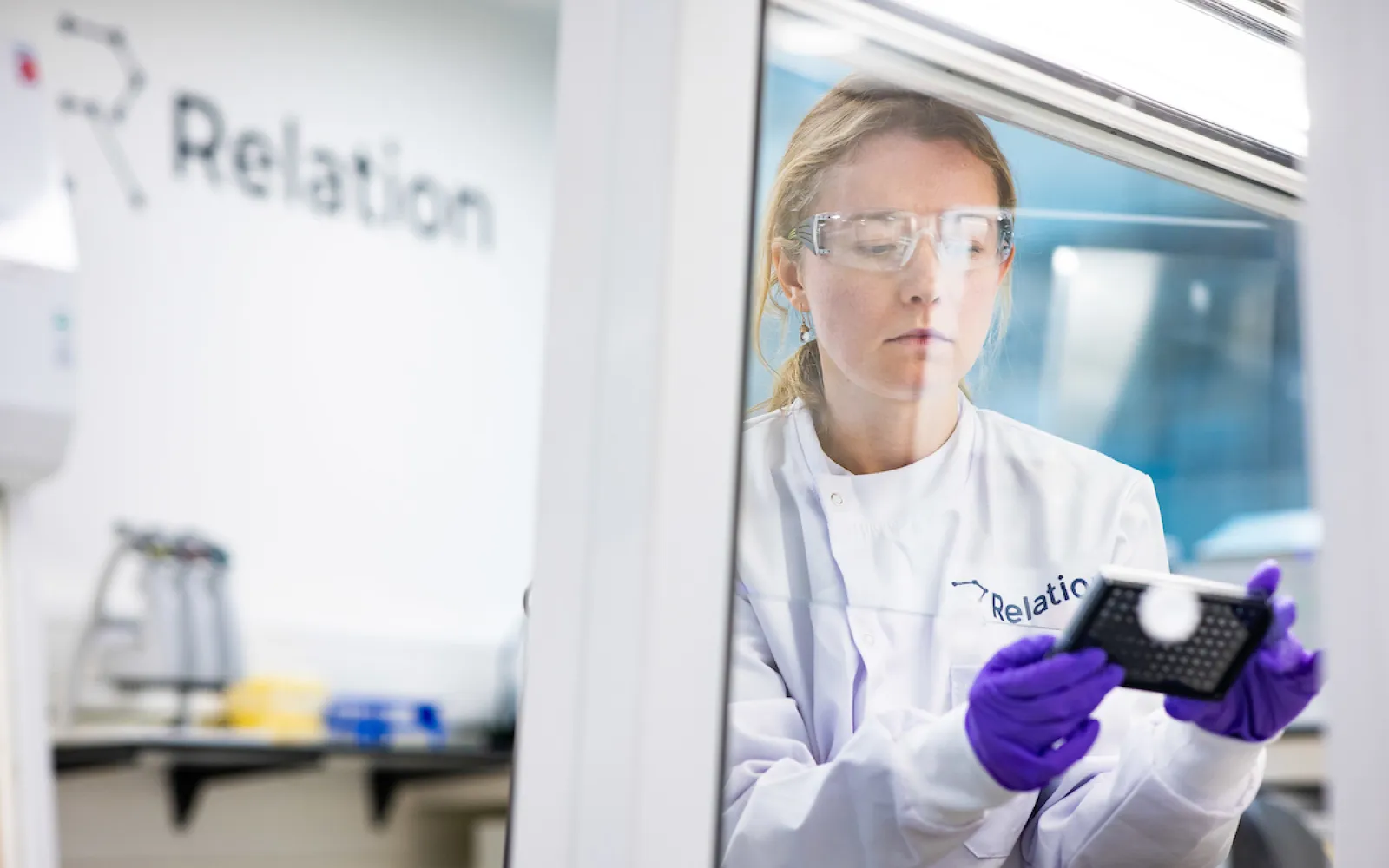
Relation
Using machine learning to understand the biology underlying disease
The just-released 2024 edition of the DCVC Deep Tech Opportunities Report explains the guiding principles behind our investing and how our portfolio companies contribute to deep tech’s counteroffensive against climate change and the other threats to prosperity and abundance. Four of the opportunities described in the report relate to computing; this is the first.
The story of AlphaFold, the AI program from DeepMind that transformed computational biology beginning in 2018, is usually framed as a victory for deep-learning algorithms. The problem it solved — predicting a protein’s 3D structure based solely on its amino acid sequence — was long considered intractable, but the AlphaFold team showed that it could be cracked using a form of attention-based transformer.
What’s sometimes left out of the story, however, is that AlphaFold was trained to recognize plausible structures using public data banks of 170,000 proteins with known structures, derived through laborious crystallographic analysis and other methods over many decades. “Can you train AlphaFold on suppositions? No,” observes DCVC co-founder and managing partner Matt Ocko. “A huge volume of X‑ray crystallography had to exist before AlphaFold worked at all.”
Steve Crossan was the first product leader of the AlphaFold team at DeepMind and is now an operating partner at DCVC. He says AlphaFold succeeded by combining the right AI models with the right data — but that in the deep-learning game, it’s ultimately the data that’s more precious. “The technique is not super hard to copy. It tends to be the case that the model, once it’s published, becomes commoditized very quickly,” Crossan says. “So if you do have a source of proprietary data — especially if you have a compounding source of proprietary data that is going to get better as your product does better in the world — then that is a sustainable advantage.”
Several DCVC portfolio companies exemplify that kind of compounding. One is Relation Therapeutics. The company’s specialty is combining models and new data to find biological targets for drugs that treat osteoporosis and other polygenic conditions (those involving mutations across many genes). It does that using a circular workflow it calls “lab-in-the-loop.” Relation sequences the genomes and RNA transcriptomes of single human bone cells from healthy and sick patients. That data feeds into active-graph machine-learning models, which predict which gene variants put people at highest risk for the disease. Then the company uses CRISPR to knock out those genes in new cell lines, singly and in pairs, and quantifies how the changes affect bone mineralization— a marker of osteoporosis. That, in turn, helps Relation’s researchers zero in on interventions that might modify the course of the disease. The company’s AI models are smart, but it’s the lab data Relation is gathering that makes them effective.
Pivot Bio, which DCVC seeded and helped launch in 2014, shows how data reigns supreme in another field, agriculture. The company sells nitrogen-fixing microbes for corn, wheat, and small grain crops called PROVEN 40 and RETURN. They’re composed of microbes that, when applied at planting, find their way into the rhizomes of crop roots and begin turning atmospheric nitrogen into nitrogen accessible to the plant, drastically reduces a crop’s need for expensive and polluting synthetic nitrogen fertilizer. The company created the additives by mapping trillions of natural soil microbes to find those with the genes needed to fix nitrogen directly. It tried billions of ways of editing those microbes’ genomes, generating a massive dataset that helped isolate the specific edits that disabled natural braking systems and further enhanced nitrogen fixation.
“These guys know more about the genomes of their species of soil bacteria than anyone on the planet,” says Zachary Bogue, co-founder and managing partner at DCVC. “With computational breeding, they can pack millions of years of evolution into a few tweaks.”
In sum: the surprising leaps forward in the power of AI models in 2022 and 2023 shouldn’t lull anyone into forgetting the years of effort and billions of dollars that went into creating and curating the data used to train them. Those algorithms understand only as much as we tell them — which is why proprietary data is and will remain king.