
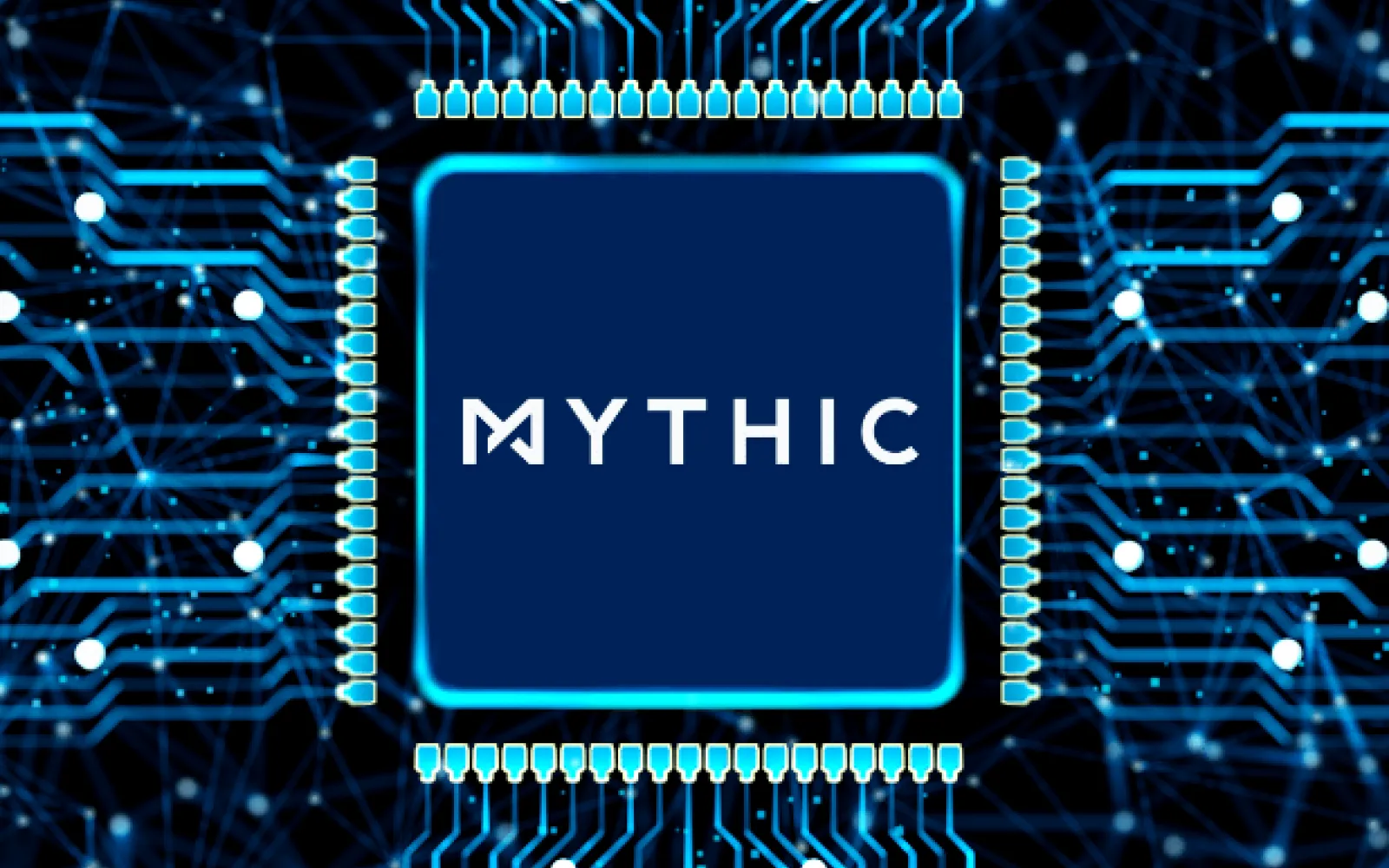
Mythic
Power-efficient AI hardware for distributed devices
The 2024 edition of the DCVC Deep Tech Opportunities Report explains the guiding principles behind our investing and how our portfolio companies contribute to deep tech’s counteroffensive against climate change and the other threats to prosperity and abundance. Four of the opportunities described in the report relate to computing; this is the third.
Between 2010 and 2020, the major data center operators held power requirements roughly flat through energy-efficiency improvements and renewable energy purchases. Then came AI’s great breakthrough in the form of large transformer-based “foundation models” such as GPT‑3, which entailed a shocking increase in electricity and cooling requirements. By 2030, according to projections from Boston Consulting Group, data centers running generative AI models could be sucking up 7.5 percent of all electricity in the U.S. — as much power as is used by 40 million homes.
The essence of the problem is that AI-focused data centers use specialized graphics processing units (GPUs) and tensor processing units (TPUs), which have more processing cores than traditional central processing units (CPUs) and consume 10 to 15 times more electricity. Newmark, a research firm that studies commercial property markets, says that as “hyperscalers” such as Amazon, Google, Meta, Microsoft build more AI-focused data centers, total data center energy demand is likely to rise from about 17 gigawatts of power in 2022 to 35 gigawatts by 2030. In a world where nations are getting serious about decarbonization, AI will be competing for that electricity with battery-powered vehicles, hydrogen production, new industrial facilities, and many other needs.
The situation demands innovation along two tracks. First, we must keep building clean new generating capacity, in the form of both variable, renewable sources like wind and solar power and 24⁄7 firm power from sources like nuclear, geothermal, and grid-scale storage. Second, as part of any vision for the responsible, ethical rollout of new AI tools, we need a plan for limiting and eventually reducing the energy requirements of foundation models themselves. The AI companies’ current strategy for improving foundation models is to keep feeding them larger and larger amounts of training data. They must also invest in techniques that reduce their models’ training requirements, such as transfer learning (using knowledge gained from one task or domain to perform better on another).
At the same time, we’d like to see semiconductor manufacturers work harder to optimize next-generation GPUs and TPUs for energy efficiency. The same goes for in-device chips, since we’ll need to offload more AI inference tasks to edge devices such as laptops and phones. Here, a company in the DCVC portfolio called Mythic is one of the leading players. Its analog silicon architecture combines processing and memory on a single chip, making it thousands of times faster and more energy-efficient than traditional digital chips. The company’s technology may ultimately be the only affordable option for running high-performance computer vision models or large language models on distributed devices such as smart-city cameras or smart-home appliances.
The hyperscalers themselves must also stop relying on renewable energy certificates to offset their consumption of electricity generated from fossil fuels, and instead find around-the-clock sources of carbon-free electricity. Microsoft’s plan to buy the entire generating capacity of a rebooted Three Mile Island Unit 1 from the mothballed plant’s owner, Constellation Energy, is just such a step — and a harbinger of the future.
To date, companies have built larger and more powerful AI models without much consideration for their training costs or carbon footprints. Now it’s time to work on making AI more inherently carbon-friendly. As information systems scholars Thomas Davenport and Ajay Kumar wrote in Harvard Business Review in 2023, “Generative models in particular…need to become greener before they become more pervasive.” We’ll look to all our portfolio companies, present and future, to do their part.