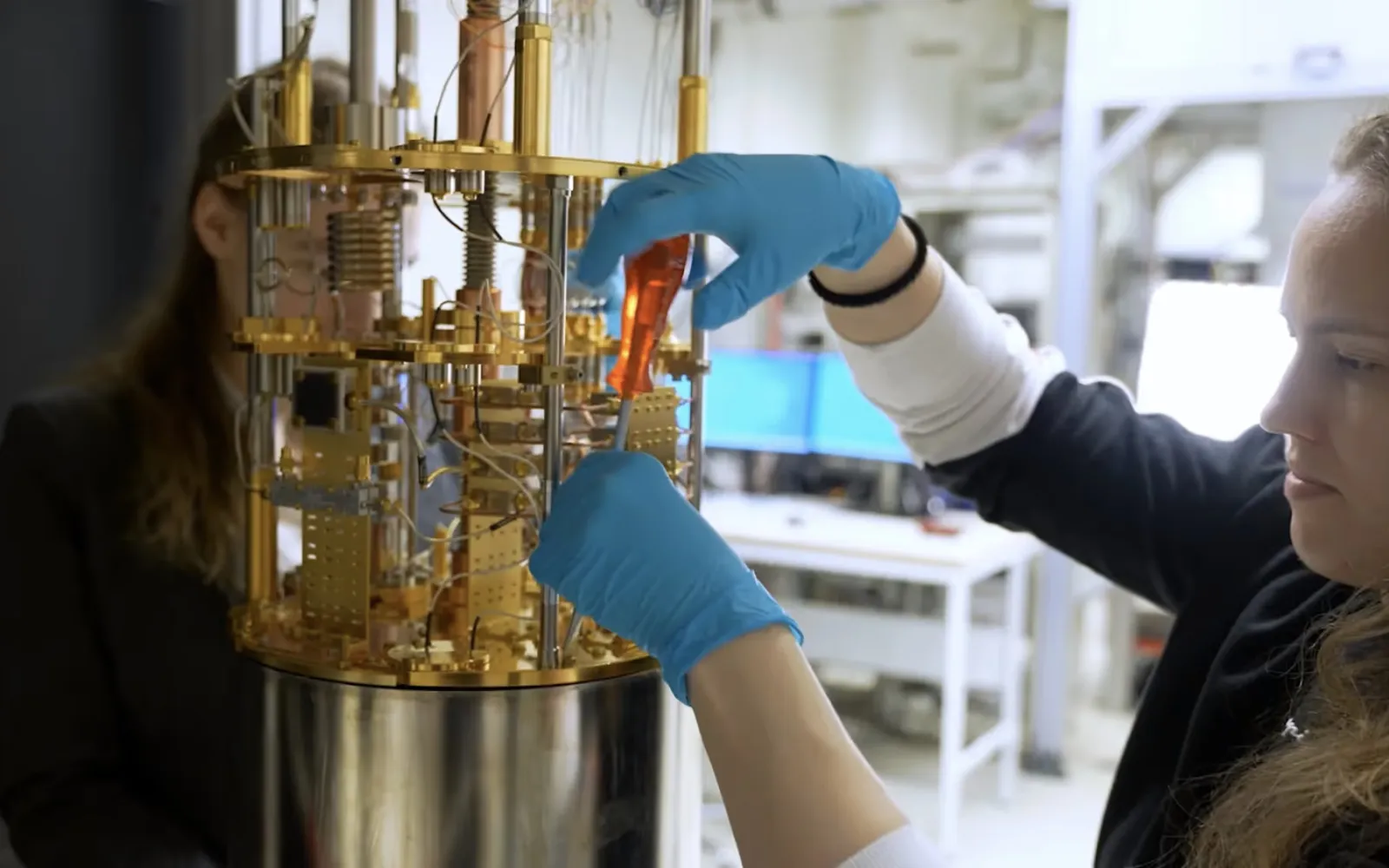
In Depth: Steve Crossan, DCVC, on AI
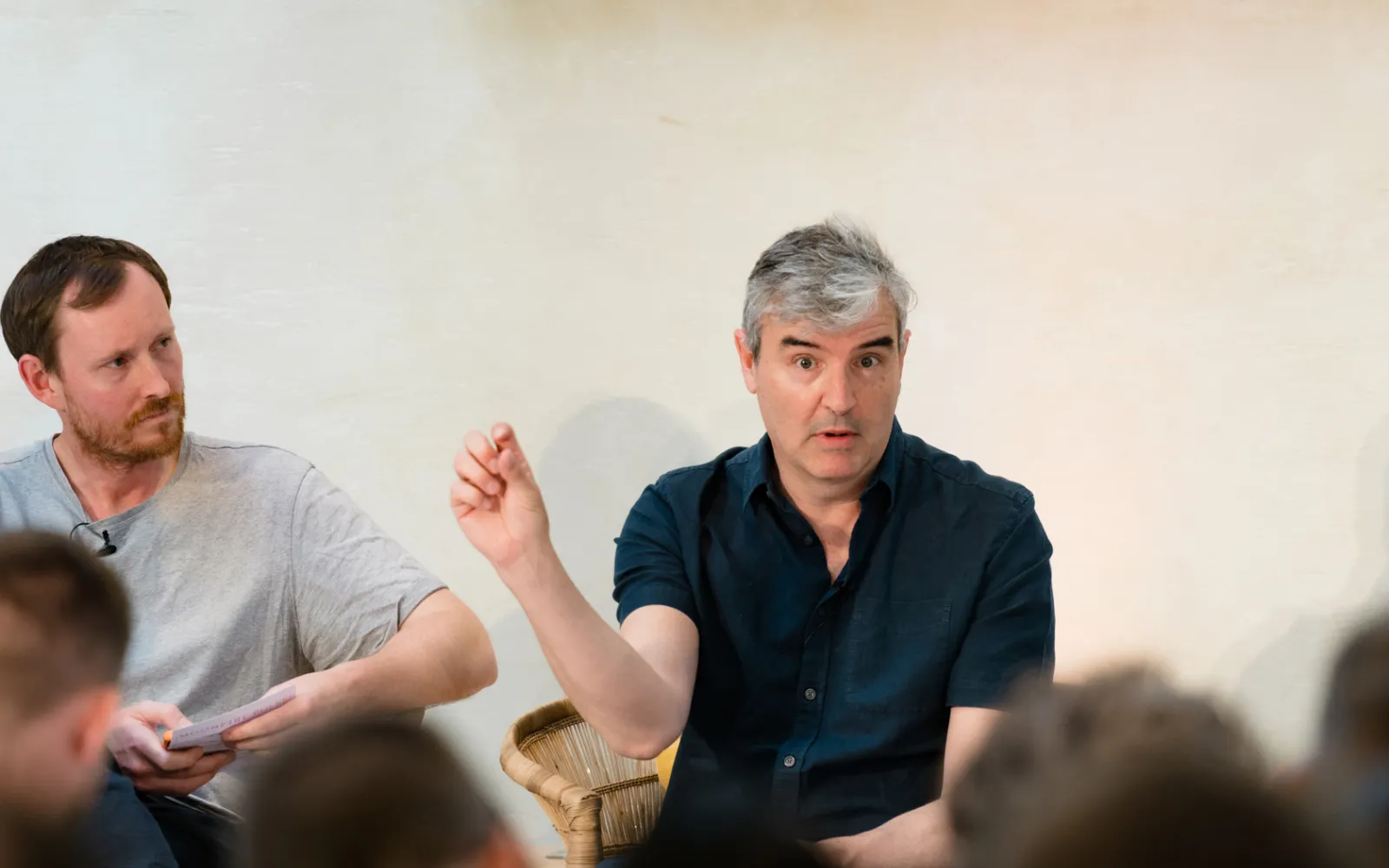
For 13 years, deep tech has been at the heart of DCVC’s investments — and at the heart of deep tech has been artificial intelligence. From the beginning, we have backed companies that use AI’s power to open new solutions for hard, often old problems. AI has helped Capella Space provide the world clear imagery of across the globe, 24⁄7, in any weather; Relation Therapeutics develop a novel approach to drug discovery; and Pivot Bio create a clean, energy-efficient replacement for synthetic nitrogen fertilizer.
But while AI is not new, the recent dramatic improvements in large language models (LLMs) are indeed a giant step forward — and have made AI’s power viscerally comprehensible for millions of people for whom it was largely a distant abstraction. Since the release of ChatGPT in November 2022, there has been a surge in both the usage of generative AI technologies and the number of platforms leveraging them. Powered by LLMs, these technologies have surprised even their creators with what they can do.
To make sense of the profound implications of this advance, we spoke with DCVC Operating Partner Steve Crossan. Before joining DCVC, Steve was VP of Artificial Intelligence and Machine Learning at pharmaceutical company GlaxoSmithKline. He also spent a number of years at Google; after Google acquired British AI research laboratory DeepMind in 2014, Steve led the team tasked with bringing DeepMind’s technology into Google products.
In this interview, Steve shares his perspectives on interpretability, the theoretical and practical limits to large language models, and the potential impact of these advancements on venture capital, company creation, and innovation.
DCVC: Google’s AI learned Bengali from just a few queries, without being told to do so, and no one knows how. We also recently learned about a provocative paper from researchers at Microsoft in which they claim AI is showing signs of human reasoning. Do we actually know what these transformers are doing?
Crossan: That is the question of the hour, and I think the answer is, “only partially.” Indeed, many of their capabilities have surprised even their creators. They can perform chain-of-thought reasoning, few-shot learning, translation, chemistry benchmarks, joke explanation, and joke writing. Initially, they were designed to predict the next word, but now they are trained as question-answering and instruction-following tools.
The field of interpretability is actively studying how transformers achieve their tasks, and we are gaining insights into their workings. At scale, transformers build representations of the world based on the data they’ve processed, incorporating knowledge structure and concept relationships. These representations serve as effective tools for reasoning and knowledge, enabling quick learning in specific domains with fewer examples. Ongoing research aims to probe the layers of stacked representations in transformers, leading to valuable insights for alignment and guiding future advancements in algorithms and architectures.
DCVC: Is there a theoretical limit to the number of parameters in a LLM, and why does that question matter?
Crossan: While I’m not enough of an expert, it seems that we don’t have a definitive answer regarding a theoretical limit to how capabilities scale with parameters and data. However, practical limits are crucial in the real world. Speculating on whether there are inherent limits to the models’ intelligence is challenging. Some believe there must be limits, but we lack a conclusive answer. The engineering challenges and costs associated with training these models are significant factors. OpenAI’s success can be attributed, in part, to their engineering focus. The cost of training GPT‑4 alone is said to have exceeded $100 million, and scaling further poses cost and engineering challenges. However, as the ever-decreasing cost of compute resources makes it cheaper to train models of a given size, it becomes essential to contemplate the theoretical limits and the possibility of leveraging this trend to develop even larger models.
DCVC: Might a transformer develop a theory of mind? If it did, what would that allow one to do?
Crossan: This is a complex and philosophical question without a definitive answer. Some researchers argue that these models can pass tests indicating a theory of mind, but theory-of-mind researchers tend to disagree. The lack of a widely agreed-upon definition or test for theory-of-mind makes it challenging to assess. In the past, the Turing test was considered significant, but now it may be insufficient as newer AI models can pass it. There are alternative tests, like the Garland Test [also known as the Ex Machina Test], proposed by novelist Alex Garland, which focuses on whether a machine can persuade someone it is conscious rather than merely pretending to be human. Overall, it remains unclear whether we can definitively determine if these models possess a theory of mind due to the absence of widely accepted tests in this domain.
DCVC: But could it be said that these transformers have something close to a model of the world, at least in certain narrow contexts?
Crossan: It seems reasonably clear that the larger models do have some kind of internal representation of the world. An example is the ability to reason over questions like, “Given a book, some eggs, and a pen, how would you build a platform to stand the pen on its end?”
DCVC: We’ve seen deep learning programs invent surprising winning moves in chess or Go. Could one invent an experiment with a surprising result?
Crossan: Deep learning programs have demonstrated surprising winning moves in games like Go and chess, which are perfect information games with known rule spaces. However, designing an experiment with a surprising result that advances science is a different challenge. There is no a priori reason to rule it out, and it’s possible that future models, including those supplemented by physics models, like Stephen Wolfram has proposed, could suggest previously unexplored experiments that contribute to human knowledge.
DCVC: If summoning the demon of a superhuman intelligence is a misplaced fear, what should we worry about?
Crossan: The debate around safety is divided between theoretical scenarios of a realized superintelligence with its own agency and internal goals, and the risks posed by bad actors who have access to powerful technology. The latter, involving misuse of technology by bad actors for purposes like misinformation, election interference, and operations at scale, are very real and significant dangers. These risks have become more pronounced with the increasing power and affordability of these tools. While the long-term concerns of superintelligence should not be dismissed, it is important to pay attention to the immediate dangers and the potential unintended consequences of powerful technology. Safety and alignment research, which is related to interpretability, is crucial but often underfunded compared to achieving the next milestone.
DCVC: What do transformers mean for VC, company creation, or innovation more generally?
Crossan: Transformers and the advancements in AI technology will reshape the tech landscape, creating opportunities and threats. This will lead to the creation of new companies and the potential for significant value generation. However, distinguishing valuable ventures from the vast number of companies incorporating generative AI will be a challenge for venture capitalists. There are already real business opportunities and money being made, especially in areas like integrating proprietary data into language models and fine-tuning models for specific purposes. Tools for non-experts to fine-tune models will emerge, affecting many software-as-a-service (SaaS) sectors. Scientific software, in particular, will undergo exciting transformations. While transformers will be a core component, the coupling of transformers with other systems, such as physics models or knowledge graphs, will drive interesting developments in the coming years.
DCVC: Many startups have been using branches of AI other than LLMs (such as machine learning or computer vision) for years. Will their work be affected by advances in LLMs?
Crossan: One interesting thing about the transformer architecture is that it seems to work across many different modalities, not just text. So, I think that other domains such as speech and vision are at least going to find something to learn in these architectures. And we’re recently seeing great examples of multimodal models which can generate both text and images, for example.
Related Content
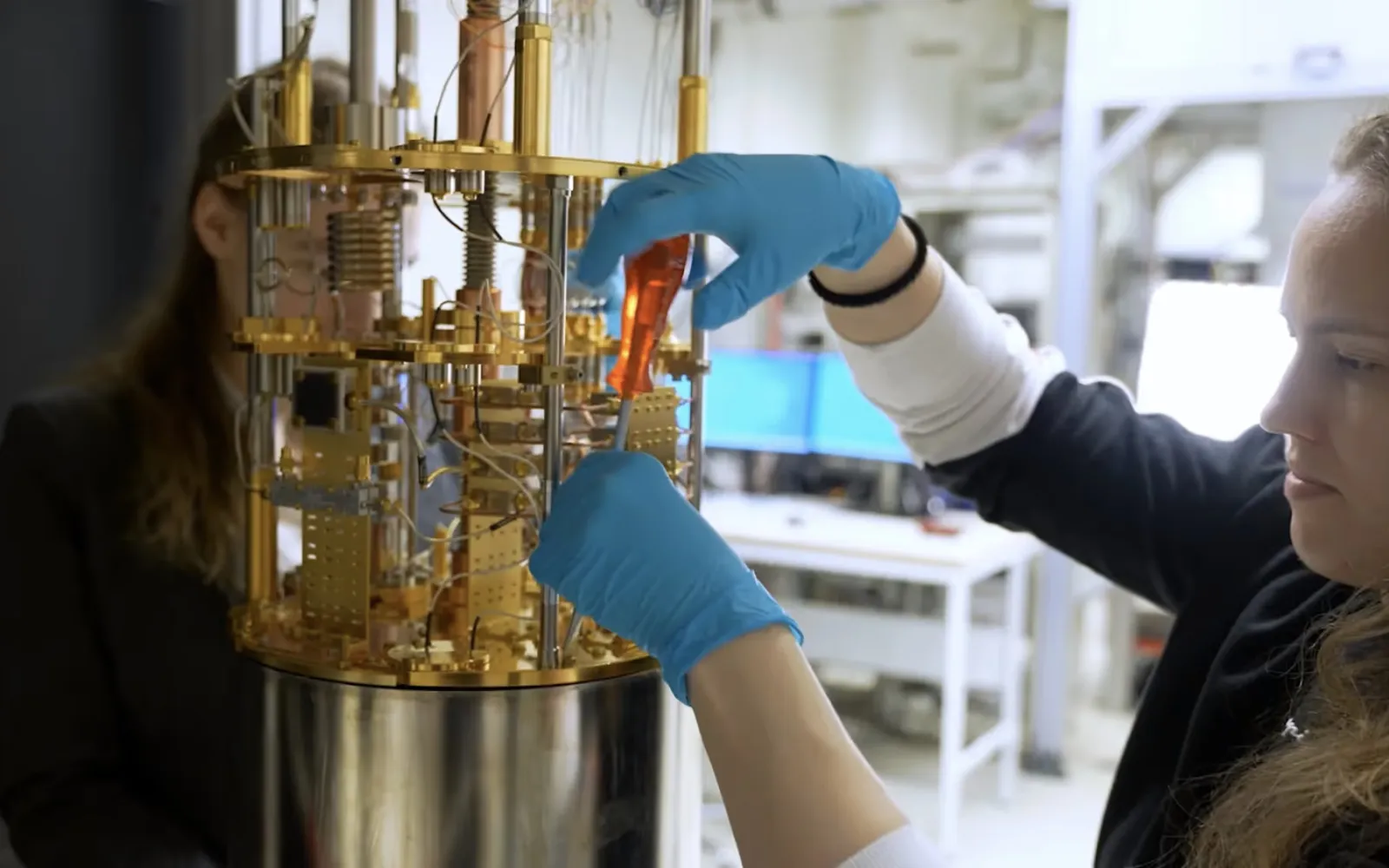
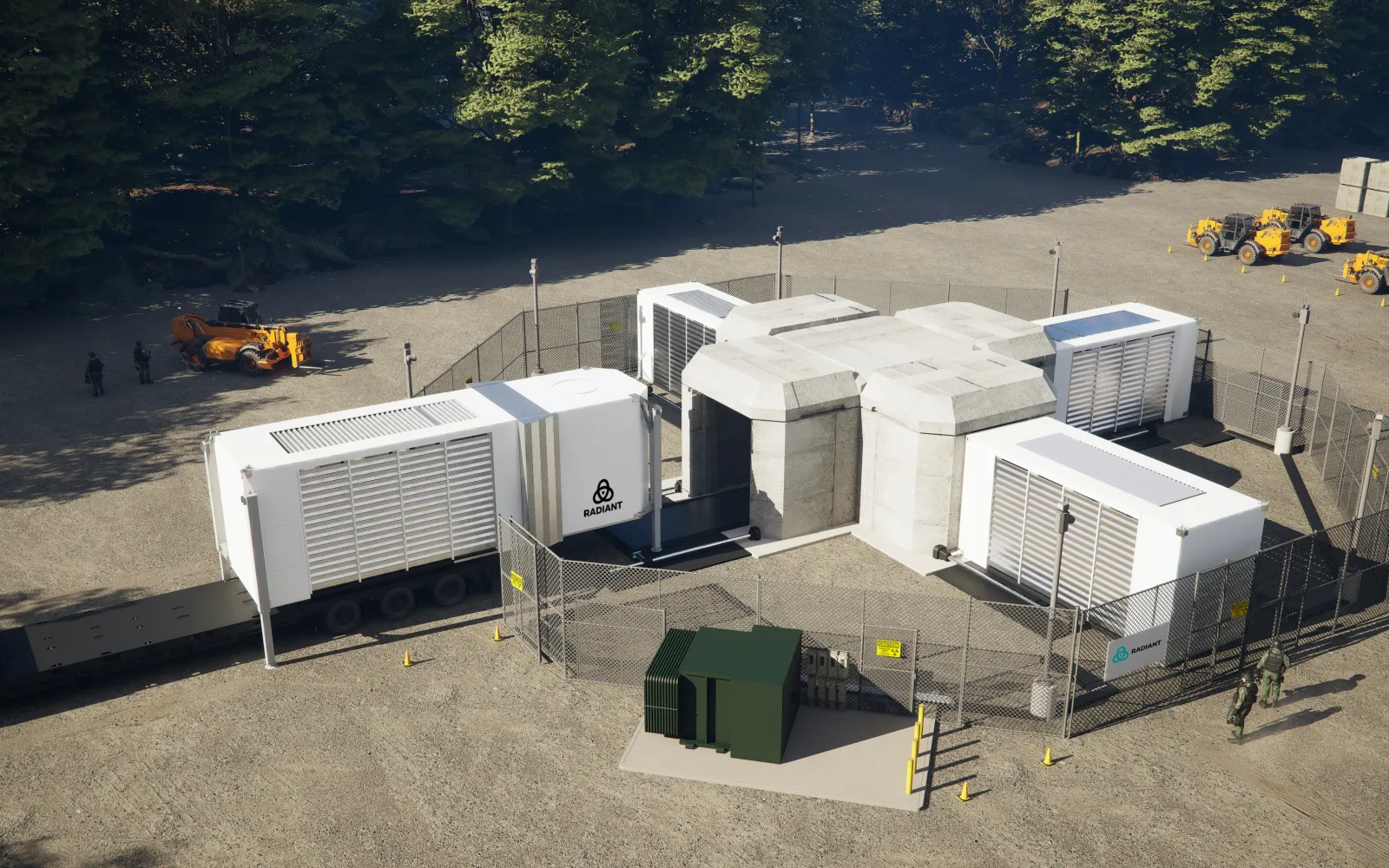
Radiant Industries raises $100 million DCVC Climate–led Series C to speed microreactors to market
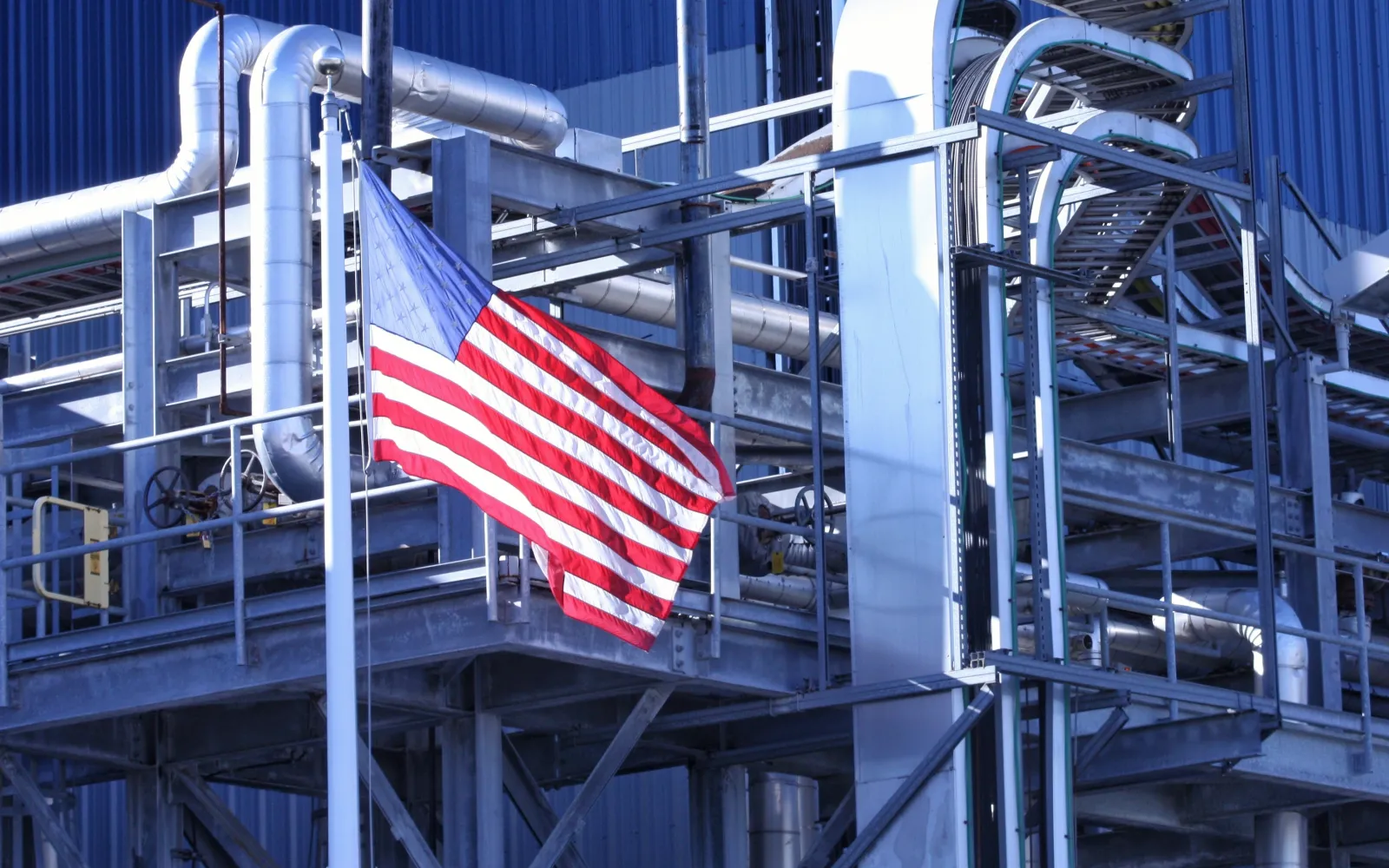
Climate tech’s lemons-to-lemonade moment: how the private sector can ensure a durable, clean American industrial renaissance
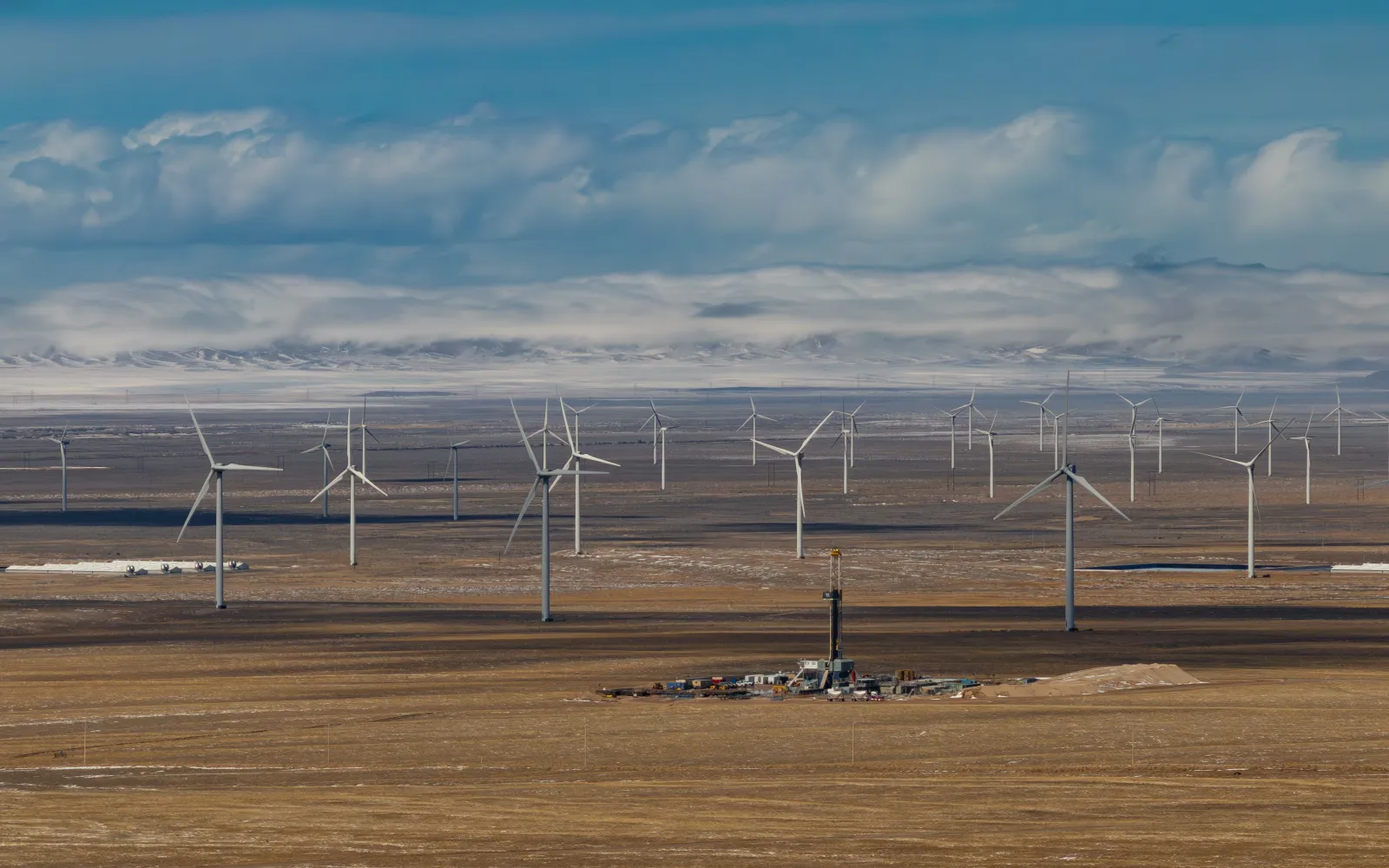